What if we could reduce premature deaths by half?
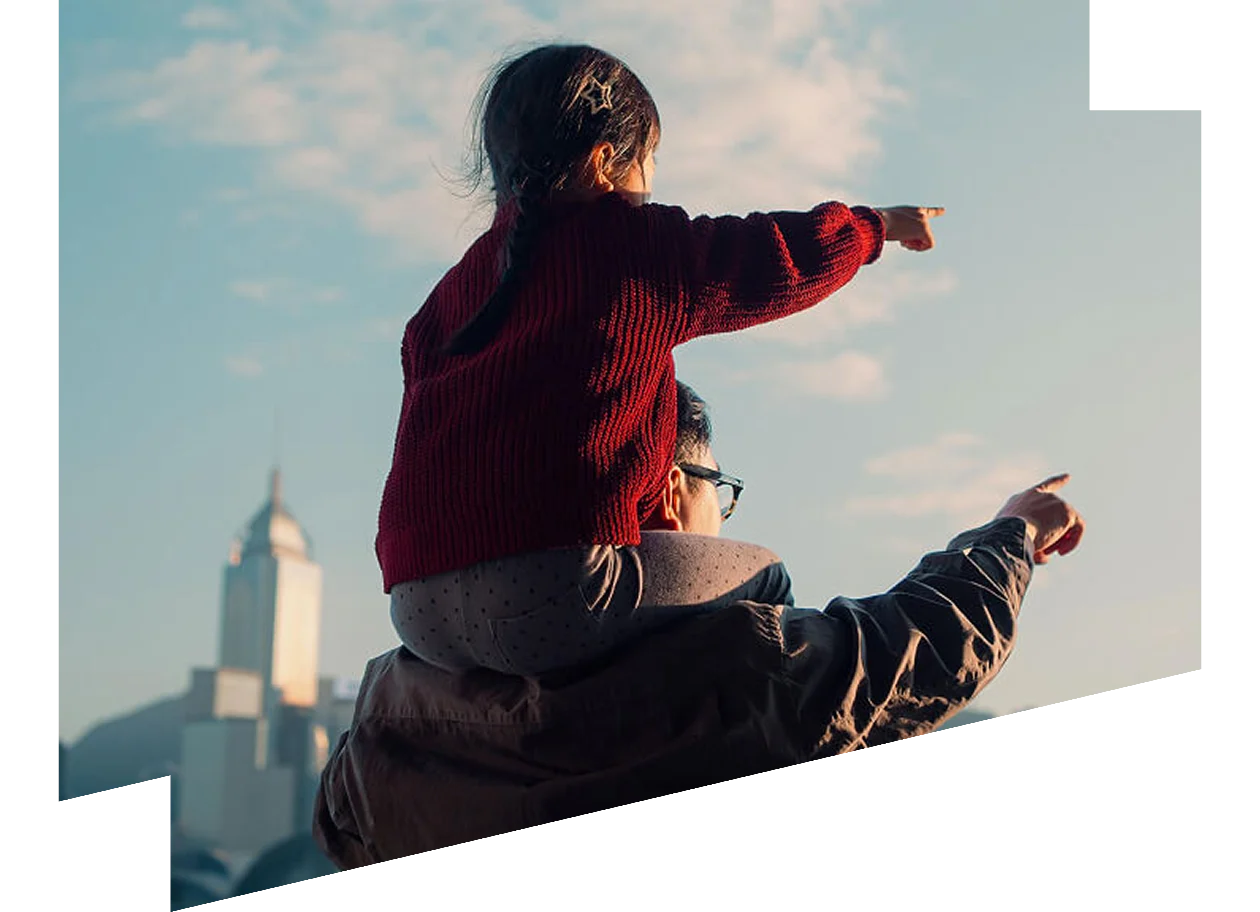
Advancing human progress together starts with big questions, fresh ideas, and the relentless pursuit of better outcomes.
Join us as we share the science, insights, and solutions that explore what it will take to make this ambition a reality — together with research and healthcare communities.