Enhancing research integrity through advanced technologies and expert oversight
How our tools and teams have evolved to safeguard the integrity of scientific research
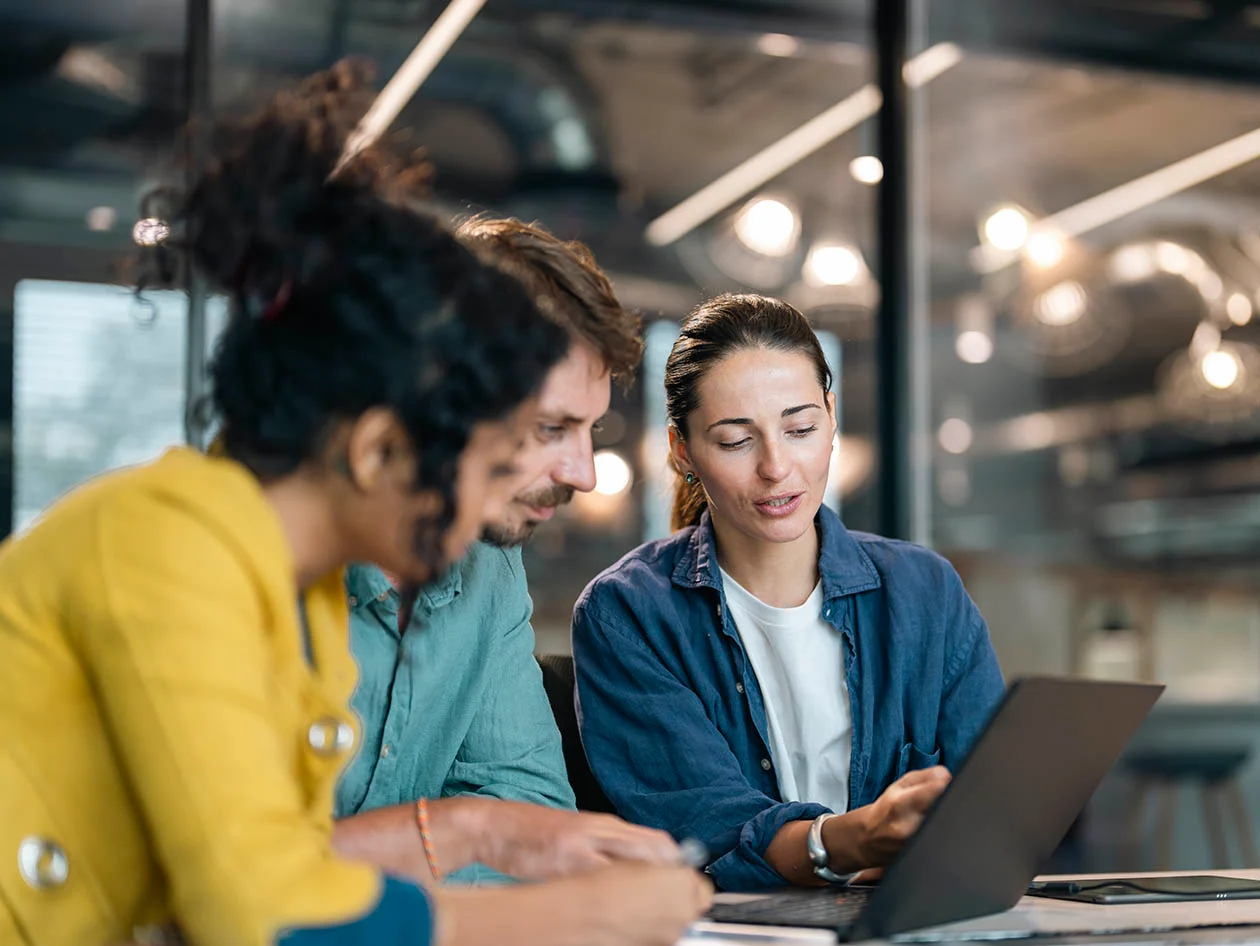
Unfortunately we don't fully support your browser. If you have the option to, please upgrade to a newer version or use Mozilla Firefox, Microsoft Edge, Google Chrome, or Safari 14 or newer. If you are unable to, and need support, please send us your feedback.
We'd appreciate your feedback.Tell us what you think! opens in new tab/window
News, information and features for the research, health and technology communities
How our tools and teams have evolved to safeguard the integrity of scientific research
How do you harness GenAI’s transformative power for R&D while safeguarding scientific integrity? Experts share their insights and success stories.
ScienceDirect AI is a smart, integrated workflow companion that enables researchers to instantly surface, cite, compare and explore trusted evidence from deep within the peer-reviewed literature.
Two Elsevier experts share their insights on vetting genAI tools for healthcare and where they can make the most impact