Does minimalism have a place in research
Minimalism is a growing trend in life and work — but can researchers benefit from the approach of “less is more”? We asked two early-career researchers recently and was surprised by what they had to say.
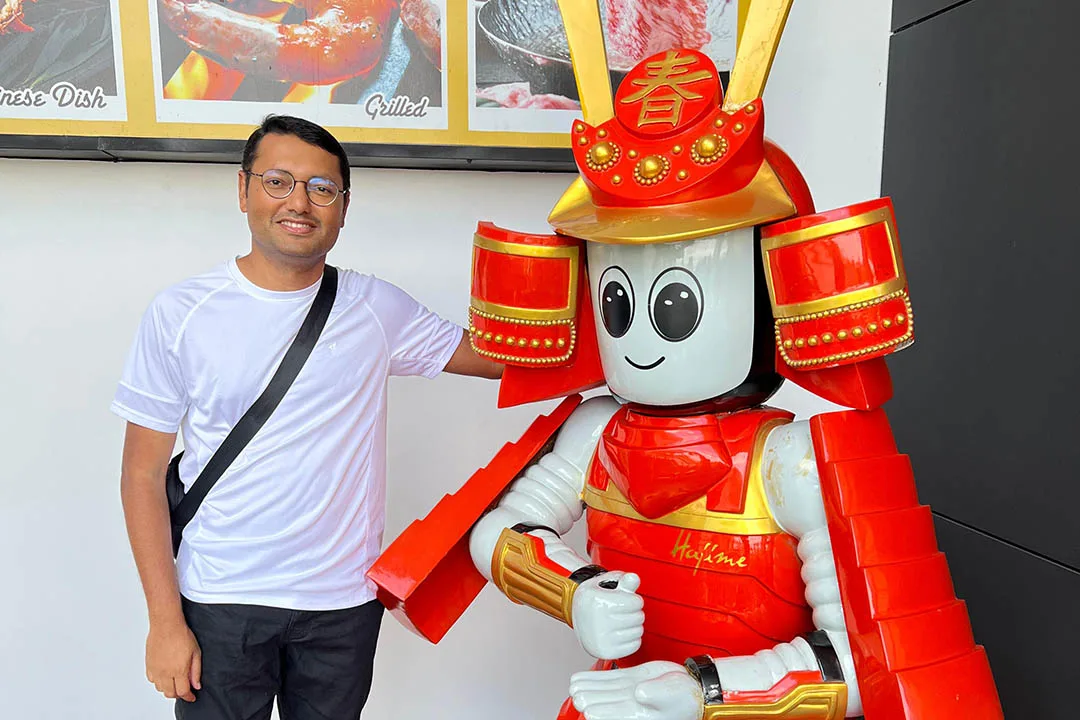
Unfortunately we don't fully support your browser. If you have the option to, please upgrade to a newer version or use Mozilla Firefox, Microsoft Edge, Google Chrome, or Safari 14 or newer. If you are unable to, and need support, please send us your feedback.
We'd appreciate your feedback.Tell us what you think!(opens in new tab/window)
News, information and features for the research, health and technology communities
Minimalism is a growing trend in life and work — but can researchers benefit from the approach of “less is more”? We asked two early-career researchers recently and was surprised by what they had to say.
Explore how library leaders finalize an agreement’s terms and ensure a successful implementation of a transformative agreements that supports their strategic goals.
Dr Engie El Sawaf knows the difference research can make to people’s lives. She researches neuropathy, a common side effect of chemotherapy that can be so severe that patients choose to stop cancer treatment. Find out how ScopusAI provides the trusted information she needs.