Mpox Information Center: Free access to resouces
In response to the World Health Organization (WHO) recently declaring mpox a public health emergency of international concern, Elsevier’s Mpox Information Center provides free access to all mpox-related information across Elsevier’s journals, medical books and clinical resources.
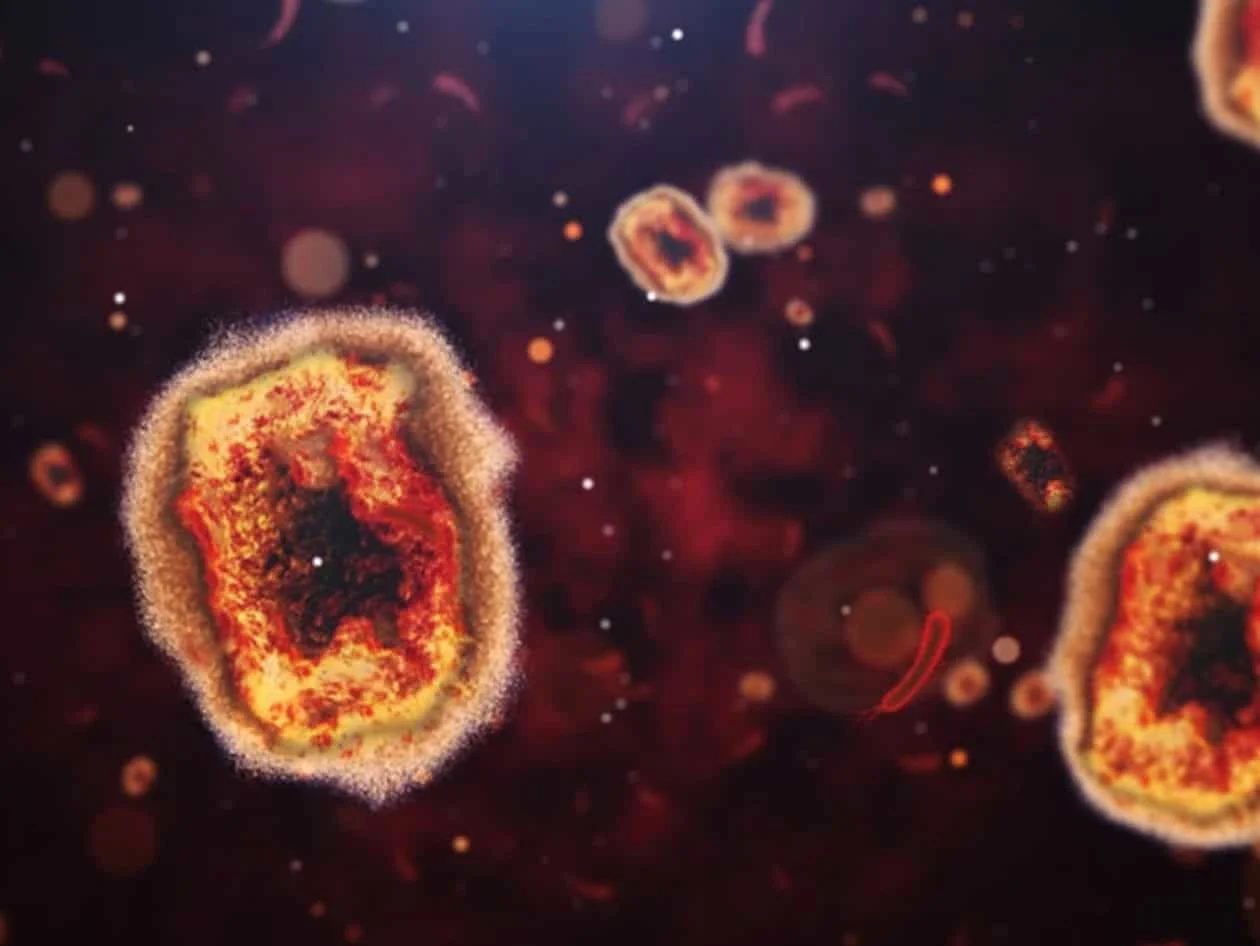