Simplify biomedical searching for all with Embase AI
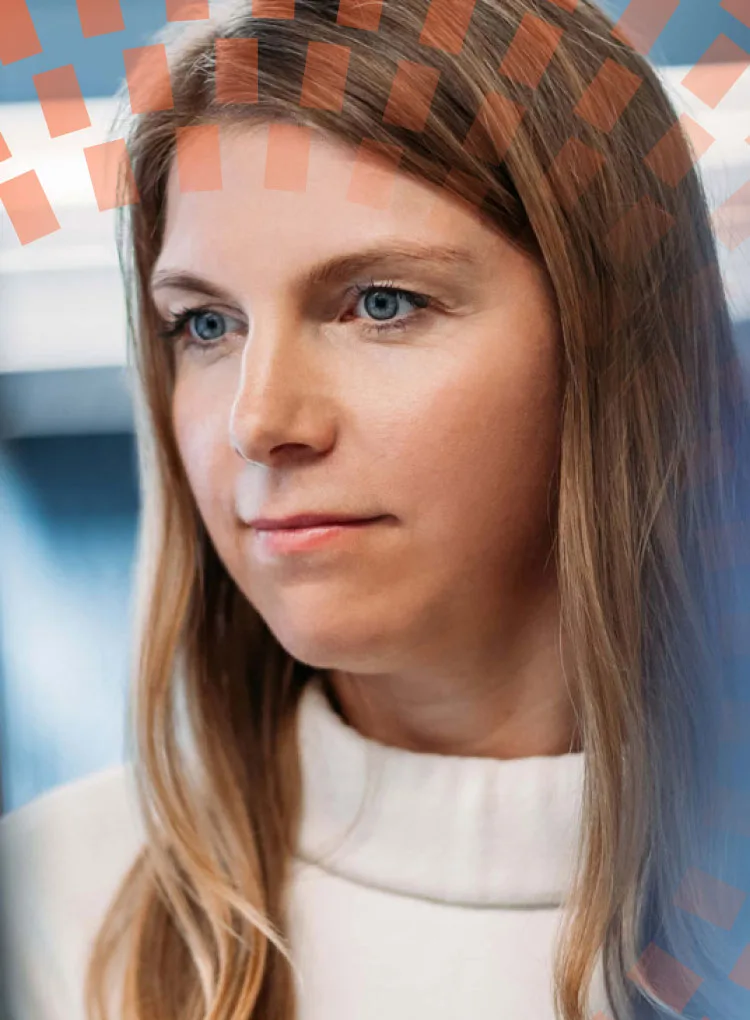
Unfortunately we don't fully support your browser. If you have the option to, please upgrade to a newer version or use Mozilla Firefox, Microsoft Edge, Google Chrome, or Safari 14 or newer. If you are unable to, and need support, please send us your feedback.
We'd appreciate your feedback.Tell us what you think!
Go further, faster in pharmaceutical R&D with connected, configurable solutions from Elsevier. Gain critical insights from trusted research and regulatory content combined with high-value analytics. Discover, innovate and develop with confidence.
Facing intense competition and growing global regulation, pharmaceutical companies trust Elsevier to transform complexity into clarity. Make informed decisions at critical moments in the innovation process based on:
Trusted life sciences information, including peer-reviewed scientific literature and regulatory data
Innovative technology that powers data transformation and analytical and predictive tools
Domain and data science expertise to solve complex problems with data solutions for R&D
Let’s shape progress together.
Explore full-text research on ScienceDirect, the leading database of peer-reviewed scientific, technical and health literature.
Accelerate drug discovery with Reaxys, a database combining over a billion chemistry data points with AI.
Conduct targeted biomedical literature searches with Embase, the premier biomedical literature database.
Identify research trends and track KOLs with the 2.4 billion cited references in Scopus.
Visualize biological relationships and filter by biological concepts with EmBiology.
Find drug safety, efficacy and DMPK data and complete approval packages with PharmaPendium.
Watch now
|
AI offers significant benefits within R&D if information integrity is paramount. Elsevier offers GenAI on top of trusted quality data to transform workflows, saving time and opening up new avenues of exploration.
Embase AI: Simplify biomedical searching for all with natural language search on trusted biomedical content
ScienceDirect AI: Instantly surface, cite, synthesize and compare trusted evidence from full-text literature
Scopus AI: Get an overview of research topics in seconds with reliable research summaries and insights
One of the biggest challenges R&D-intensive companies face is to quickly and reliably extract meaning from a vast and growing sea of scientific data. Discover a range of solutions to help you transform data into discoveries.
Power your custom applications and third-party tools with domain-specific curated and enriched Datasets
Get more from your data with text analytics and data enrichment tools from SciBite
Solve data integration and applied analytics challenges with support from the domain and data science experts on our Professional Services team
Fill out the form below to sign up for the ScienceDirect Industry Outlook, a quarterly update spotlighting some of the most important research impacting the Pharmaceuticals industry.