10 ways Elsevier is using data to drive inclusion
December 15, 2022 | 11 min read
By Anita de Waard, Harry Muncey, PhD
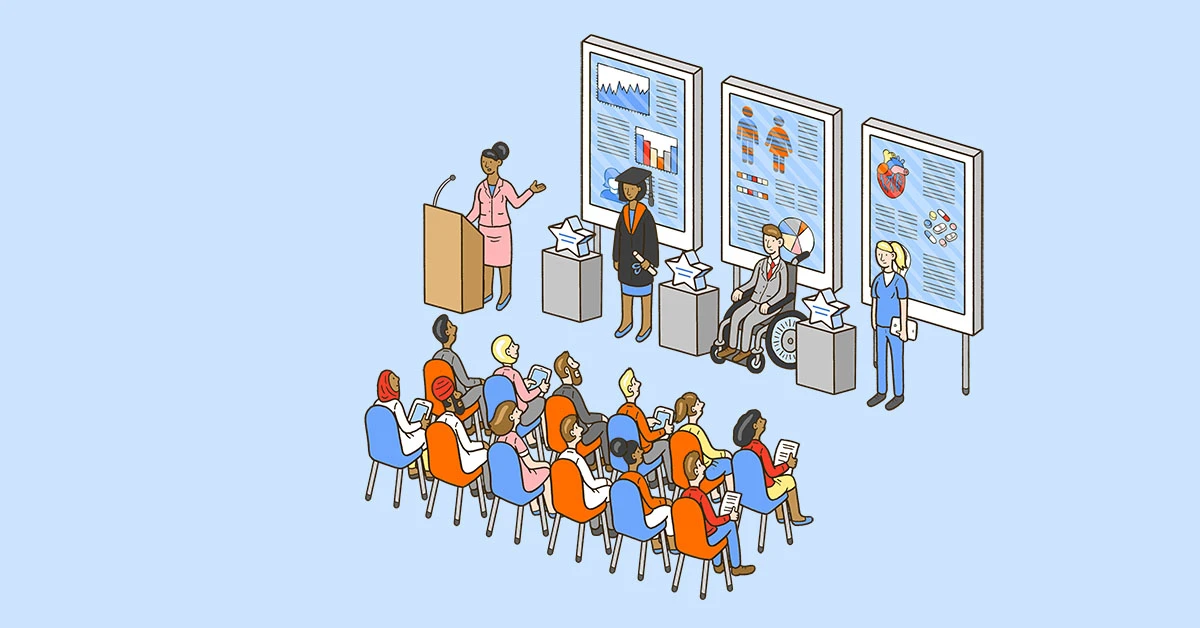
Data is helping improve representation, change systems and support early career researchers
Diversity is essential for a successful research ecosystem. When we embrace our differences — including gender identity, race and ethnicity, and age — we enhance innovation, productivity and decision-making.
Yet we know there is inequality throughout the research and healthcare communities we serve, and therefore in the data and systems they use in their work.
Speaking on a panel this year to celebrate the fifth anniversary of the Harvard Data Science Initiative, Elsevier CEO Kumsal Bayazit commented:
We look at the data to ask the questions, to compare and contrast, and to identify actions. It takes a long time; it's slow work and it’s very easy to get frustrated, but the needle does move in the right direction when you look at it through the lens of decades.
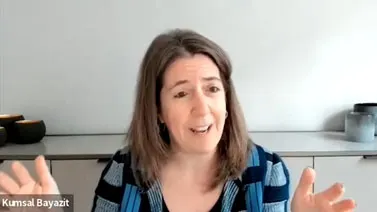
Kumsal-Bayazit
To reduce this inequality, efforts are being made across Elsevier to improve representation, educational approaches, data models, and decision support to detect bias and expand inclusivity. We are advancing inclusion in our data and systems by focusing on three areas:
Improving representation — gathering data, tracking and measuring to make a difference in all the work we do.
Changing systems — factoring in sex, gender and ethnicity into research and health education.
Supporting early career researchers — ensuring diversity and lifting up individuals.
Here are 10 examples of how Elsevier is using data science in these three areas to reduce inequality and improve inclusion and diversity in the data that underpins our systems and tools.
Improving representation
Artificial intelligence (AI) has huge potential to help people throughout the research ecosystem, from researchers who want to find the information they need to editors looking for suitable reviewers. But before AI can align with and support inclusion, the challenge of historical bias will need to be overcome.
Elsevier is using data to take steps to improve representation, whether it’s in the body of published work or in the systems that underpin it.
1. Creating an Inclusion & Diversity Advisory Board
Collaboration is key to achieving change. We are working with our network of stakeholders to influence and address inequality in the communities we serve and to promote inclusion and diversity best practices to help foster diverse and inclusive future generations of STEM graduates.
Elsevier’s Inclusion & Diversity Advisory Board was established for this purpose. Since 2020, the board has been working with research leaders, funding bodies, higher education institutions, academics and other publishers to drive inclusion and diversity on editorial boards and in peer review and scientific conferences across the STEM academic career path. The board also encourage enhanced sex- and gender-based analysis and reporting in research studies.
The board gathers inclusion and diversity data in its annual report, which highlights areas of progress and summarizes the actions being taken. The latest report shows that during 2021, Elsevier increased the representation of women on Editorial Boards of proprietary journals from 16% to 24%. Also, by the end of the year, 772 journals (43% of proprietary journals) were displaying the Editor Gender Diversity Indicator and visualization pod on their homepages.
2. Reporting on gender
The Inclusion & Diversity Advisory Board is also instrumental in Elsevier’s reporting on gender. Since 2015, Elsevier has published several reports on gender in research: a report on Germany (2015), two global reports (2017 and 2020), and a report on Portugal (2021). The data in the reports show where effort is still needed to ensure equality for women in terms of publication outputs, citations, awarded grants and collaborations.
The latest global report — Gender report 2020: The Researcher Journey Through a Gender Lens — is based on 20 years of data from Scopus across 12 geographies and all 27 Scopus subject areas. Although progress is being made in achieving equity, the report shows that women still trail men in terms of their number of publications and citations. Specifically, more women are publishing, but less so over time. Fewer women publish as first and last authors, as authors of patents, and in the physical sciences.
3. Gathering race and ethnicity data
In 2021, Elsevier and the Royal Society of Chemistry convened a series of workshops on Race and Ethnicity in the Global Research Ecosystem with a selection of research funding organizations. The aim was to explore the question: How are organizations supporting national transformation on issues around diversity, race and ethnicity?
One of the challenges identified was the collection of data on race and ethnicity. Compared to gender, race and ethnicity is a far more complicated topic to ask people about. To move the industry toward a standard way of tracking diversity in journals, Elsevier led a publisher-agnostic survey that aimed to refine a two-question race and ethnicity schema. The results are feeding into an approach that can be used by all publishers to help promote greater diversity in publishing.
This week, The Lancet published a special issue on Advancing racial and ethnic and equity in health, “bringing together the strongest evidence and analysis on advancing racial and ethnic equity in science, medicine, and health.” Throughout all these efforts, Elsevier aims to be accountable to communities affected by racism and discrimination by living up to our commitments on diversity and supporting a joint commitment for action on inclusion and diversity in publishing.
4. Adopting Responsible AI Principles
RELX, Elsevier’s parent company, has created Responsible Artificial Intelligence Principles to provide high-level guidance for anyone at RELX working on designing, developing, and deploying machine-driven insights. The guidelines provide a risk-based framework drawing on best practice from within our company and other organizations. Elsevier has robust policies and processes in place that are applicable to AI-enabled solutions, and the purpose of the Responsible AI Principles is to complement these. AI is a field that evolves continually, at unprecedented speed and scale.
These principles will iterate over time, based on colleague and customer feedback, as well as industry and legislative trends. This will allow us to be proactive, ensuring our solutions develop in line with our values, and maintaining our stance as a thought leader in the market.
5. Reducing bias in reviewer selection
One such challenge Elsevier is currently working on is the Reviewer Recommender tool. Based on datasets from Scopus, SciVal and our Editorial Manager, through which about 80,000 editors choose and invite more than 1 million peer reviewers every year, this tool is designed to help editors select people to review article submissions.
A study by Elsevier’s Submissions Data Science Team revealed bias in our recommendations. The issue comes from the fact that AI systems are built on a history of often biased human decision-making. The data science team has been adjusting the tool, including building in fairness metrics to make it more representative of communities historically discriminated against – for example, women. The context is also important: for example using fairness metrics can account for different proportion of women academics currently available to review in different disciplines.
Changing health and research systems
By factoring sex, gender, race and ethnicity into research, health and education, we can put change in motion that results in more equality in data.
6. Providing a virtual female anatomy model

Complete Anatomy's diversity vision
One approach to reducing inequality in medicine is through improving equality in health education. For hundreds of years, men’s bodies have been considered the norm or standard in medicine and health education, while women’s bodies have been treated as a “special case.” This has resulted in inequality of care as well as limitation of knowledge in the ability to accurately diagnose and medically treat women. Consequently, women are misdiagnosed more often than men. For example, women are more likely than men to be wrongly diagnosed following a heart attack or a stroke, because the symptoms present differently in female bodies.
Having virtual anatomy tools could help achieve equality between male and female bodies in medical education and ultimately patient care. Additionally, a clinician who has been trained to learn the similarities and differences of both bodies and genders will be better equipped to recognize sex-specific illnesses—for example PCOS in females and testicular cancer in males. In January, Elsevier released the most advanced 3D full female anatomy model ever available, based on four years of research with experts, on the platform Complete Anatomy. The lead subject matter expert who worked on the platform update was Dr Yasmin Carter, Assistant Professor of Translational Anatomy at the UMass Chan Medical School in the USA. She said:
One of the inherent dangers in using only the male body as ‘anatomical normal,’ and the female body as a variation, is perpetuating sexist attitudes. This unconscious bias will be carried by learners into their future interactions with the body, including potentially with patients. By giving educators the option to choose the female body as the basis for their students’ education, Elsevier is helping evolve equitable teaching practices by normalizing the female body.
The tool was featured on the BBC this year.
7. Supporting inclusion in nursing care
Nurses interact with all sorts of people, and it’s important that patient diversity is reflected in their education — including online. Elsevier’s Shadow Health platform enables nurses to practice interactions with patients in virtual settings.
The simulations are available for students in a variety of programs and at different levels of expertise to practice their nursing skills in a safe and standardized environment. They can interact with patients with different cultural backgrounds, race and ethnicities, gender identities and body shapes, for example. They can also practice providing care in sensitive scenarios, such as suicide prevention, without impacting possibly vulnerable populations.
8. Supporting datathons in pharma
Data is also key to ensuring equity and inclusion in the development of new drugs. Elsevier is collaborating with pharmaceutical companies to make data, knowledge graphs and software available for bioinformaticians to support open-data drug discovery and repurposing efforts.
This collaboration takes the form of datathons: events where participants can showcase their data expertise. For example, work done by the Discovery Lab in Amsterdam — a joint public-private partnership with two Amsterdam universities — was used in a datathon developed with Astra Zeneca to find new epigenetic targets to treat cancer. Data also helps build teams: Elsevier and Pistoia organized a different Datathon on Drug Repurposing for Rate Diseases, with an added goal to find new analysts to work on this topic.
9. Cultural Insensitivity Prototype
In 2021, our Data Science and Clinical Analytics group in Data Science Health developed a prototype to programmatically mine through medical content with the goal of identifying terms that may perpetuate systemic racism and biases across our current flagship offerings in health education. The Natural Language processing/machine learning model was trained to pull content based on gender, culture, age, religious affiliation, sexual orientation, and disability biases from our various content types in production, thereby reducing bias throughout our flagship products.
Supporting early career researchers
To ensure the next generation of researchers and medics work in an inclusive and diverse research ecosystem, we need to support early career researchers. By ensuring diversity is considered in education and recruitment opportunities, Elsevier is helping create an inclusive environment.
10. Developing a recruitment dashboard
Although representation is improving in research, equity has not been achieved, and diversity is a work in progress. Recruitment plays an important role in this. Elsevier’s International Center for the Study fof Research (ICSR) team has been collaborating with Queensland University of Technology, Australia, to design a tool that counters bias in academic recruitment.
The tool uses high quality and consistent data to shortlist candidates for research-centric roles. It was successfully used alongside expert review to support the selection of Australia’s 2021 Prime Minister’s Prizes for Science. The tool uses a multidimensional set of metrics to reflect achievement in research, leadership, collaboration, mentorship and public engagement of a candidate using data from Scopus, SciVal, PlumX Metrics, PatentSight (from Elsevier’s sister company LexisNexis) and NamSor.
As filters are applied against these indicators, the effect on the (inferred) gender of the candidate pool is made clear, highlighting unconscious bias and “nudging” users to consider what they really value. In this way, it highlights inherent biases in current practices, such as the reliance on existing personal networks in academic recruitment.
In summary, there are a great many efforts across Elsevier to drive inclusion, diversity and bias using data. We are committed to working on these topics and related efforts and are looking forward to collaborating with the research community to reduce bias and work towards a more equitable, fair and inclusive scientific workplace.