Conferences
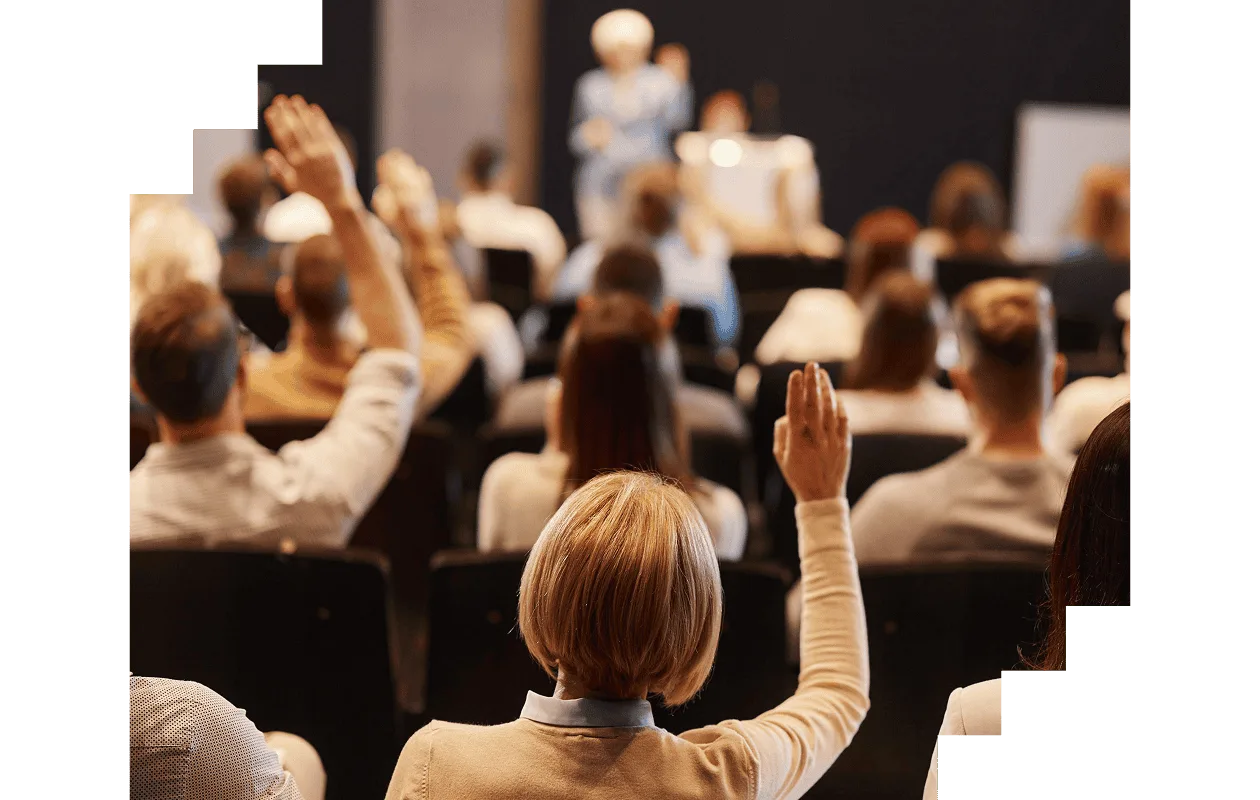
We organize a variety of international conferences covering Life Sciences, Physical Science & Engineering, Social Sciences and Health Sciences.
Unfortunately we don't fully support your browser. If you have the option to, please upgrade to a newer version or use Mozilla Firefox, Microsoft Edge, Google Chrome, or Safari 14 or newer. If you are unable to, and need support, please send us your feedback.
We'd appreciate your feedback.Tell us what you think!
Come and meet experts in your field and enjoy world class content at one of the many events that Elsevier proudly hosts or participates in every year.
Overview of the conferences, exhibitions and webinars that Elsevier organizes or participates in.
We organize a variety of international conferences covering Life Sciences, Physical Science & Engineering, Social Sciences and Health Sciences.
We participate in several hundred exhibitions every year. This allows us to personally meet our customers, editors, authors and show case Elsevier products.
View exhibition landing pages and check our calendar of events. Find our booth, browse article selections, journals and more.