Today’s innovations are built on organized data
November 6, 2023
By Ann-Marie Roche
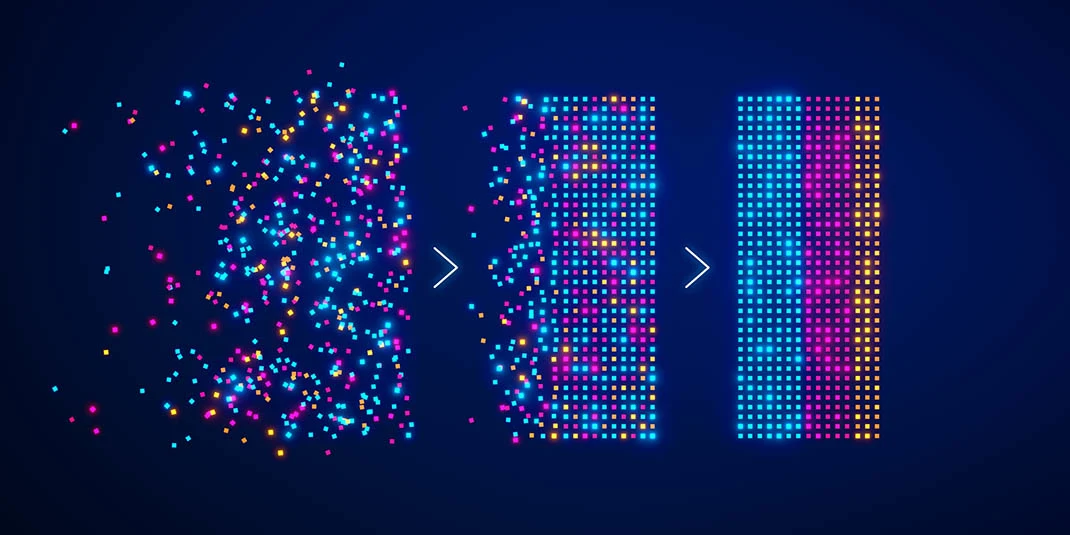
SciBite leader Dr Joe Mullen will join AI and data experts in the upcoming webinar “The perils and pitfalls of generative AI for R&D.”
While generative AI is taking the world by storm, a more fundamental aspect of data science excites Dr Joe Mullen even more.
“AI technologies will come and go, but foundational data management is forever,” he says. “Having your data in order buys you the agility to quickly jump on and reap the benefits of the latest innovations — whether it’s around machine learning, LLMs or beyond.”
Joe is Director of Data Science & Professional Services at SciBite opens in new tab/window, a semantic analytics software company acquired by Elsevier in 2020. He will be among four data science and AI experts on a free webinar opens in new tab/window Wednesday for the pharmaceutical industry.

Joe Mullen, PhD
Focus on the problem
“We’re strong believers that data fuels discovery and we’re always out to apply the latest tech applications to help accelerate scientific breakthroughs,” Joe says.
“Of course, it can’t be any old data,” he adds. “It needs to have provenance and hence be well-managed. Only then can you make evidence-based decisions to generate a hypothesis — the bedrock of scientific progress. And the data must be built on being FAIR: Findable, Accessible, Interoperable and Reusable. Then you really have something.”

FAIR data is findable, interoperable, accessible and reusable (Source: FAIR Principles opens in new tab/window; image by SciBite)
As an example, Joe pointed out that SciBite is able to support R&D in the Life Sciences for such matters as target prioritization, market surveillance, adverse event detection and drug repositioning opportunities:
Basically, our team helps customers solve their problems by getting the most out of their data. And that’s not only about expediting insight extraction, but also lowering the barriers of entry for customers to get the most of what we offer. And while we use the latest machine learning technologies to help make this happen, it’s all based on an understanding that all the best digital strategies are built on strong data foundations. And that there’s a lot of data out there waiting to be structured and mined for value.
Webinar: “The perils and pitfalls of generative AI for R&D”
Dr Joe Mullen will be among the panel of AI and data experts on a free webinar Wednesday, Nov 8 at 9 am EST. This is the first in a four-part series called AI in innovation: Unlocking R&D with data-driven AI.Experts will explore the perils, pitfalls and promise of generative AI for R&D. From poor data to the frame problem, RAG and vector-based IR, they'll outline the issues that can derail your AI projects. And they’ll also answer your questions about how Elsevier licenses, delivers and updates data for use in generative AI.
A passion fueled: it’s in the numbers
Joe says he was always solutions-driven:
I always look at problems and try to work out how best to resolve them. Initially, I was very enthused by biology — understanding how the body works. But a deep appreciation for data analytics was sparked by a small module while doing my biology degree.
This passion led him to complete a master’s degree and then a PhD:
I found it fascinating how you can take a file filled with all this human-level noise and then do something with it to identify a potential hypothesis. And today, the technology to generate such a hypothesis has developed enormously. And the way that we analyze that data is always evolving. But ultimately, our goal remains to be able to see what data can tell us in as automated and seamless a way as possible.
With a PhD in semantic data integration — developing knowledge graphs to drive the identification of new uses for existing drugs —Joe was a perfect candidate for startup SciBite: “I was hired as number 13,” he recalls. “Now six years later, we have around 80 people. It’s been very hectic and incredibly rewarding being part of this incredible data science team — a team I am now lucky enough to lead.”
A match made in structured data heaven
“We’ve always been a software company that allows customers to get the most value out of their data,” Joe says. “And since we’ve been acquired by Elsevier — who have the gold standard in data and data platforms — it’s a pure pleasure to see how our combined efforts work to provide even better solutions to those problems we see customers coming in with.
“SciBite was always small and agile. We were always able to turn left or right when we wanted to. And that hasn’t changed much. We still operate as an independent business unit. But there's a great synergy between us and great opportunities to work together. From both a technical and a business perspective, it all makes perfect sense. And Elsevier doesn’t just have data, they also have human expertise.
“And human expertise is not going to reach any sell-by date. I very much align with that expression: ‘AI is not going to replace humans, but humans with AI are going to replace humans without AI’.”
Q: What makes quality data? A: Subject matter experts
“Obviously, everybody has a lot of data,” Joe says. “Now, in order to understand that data, it takes the Subject Matter Experts (SMEs) to sort it out opens in new tab/window: to build the definitions and standards — the ontologies — so we can recognize different entities within the data, may it be a drug, a disease, a protein or a phenotype. We’ve always had a lot of SMEs in the life sciences. And now Elsevier is opening things up for us by also having SMEs in other verticals such as chemistry and engineering. They’re famous for having a lot of these SMEs.
“These are people who understand the importance of building public identifiers that build on the FAIR data principles opens in new tab/window. Yes, technologies can expedite a lot of these tasks but you need the human in the loop opens in new tab/window to validate the information.”
Data is king
The fact that SciBite retains its startup mentality dovetails nicely with the idea of having strong foundational data management. “It comes down to the fact that technologies may come and go, but your data is what remains consistent throughout. By having good quality, foundational data management, it allows you to nimbly pivot and make use of the next state-of-the-art technology when it becomes available.”
Large language models (LLMs) are a case in point. Certainly, its most publicized version, ChatGPT put data science on the map for the general public as an exciting field. However, such generalized solutions simply do not cut it in an industry based on a specialty knowledge. And while Joe admits much of SciBite’s work around organizing the data may seem dry to some, it remains fundamental. In fact, once you have your data house in order, things can get exciting fast.
“There are certain areas we can now do rather quickly — and are even developing more cookie-cutter-like approaches to. But where I get very excited are the deeper dives into scientific questions that we may not have touched on before: microbiome target identification, post-translational modification to disease linkage, and any other type of relationship inference.”

JM
Joe Mullen, PhD
Director of Data Science & Professional Services, SciBite at Elsevier
Exciting new phase
“Often, we are now dealing with deeper scientific questions that require many different lines of evidence,” Joe says. “And we’re in an exciting phase where we have the foundational components in place so we can better connect the dots between multiple data sources — may it be Elsevier’s extensive databases, customer internal databases, or those many open data sources.
“But, at the same time, every point during our customer’s R&D process, they’ll have to submit things to regulatory bodies. So you need to know exactly where you're getting these hypotheses from — where you're actually identifying this information.”
In other words, it comes down to the touchstones of science: providence, reproducibility and transparency — all current shortcomings of LLMs:
It goes beyond the hallucinations — where LLMs generate false information. There’s also the irony of OpenAI refusing to disclose anything about what went into GPT4. There are still too many issues to be sorted out.
Transparency is everything
“But this doesn’t take away from the potential of LLMs, and they are already an amazing tool for certain tasks,” Joe adds.
And down the road, he sees potential in LLMs helping lower the barrier for users to explore all the information and interrelationships that the machine learning algorithms have found.
“That will be the big play: the customer being able to interact with all these databases using natural language thanks to an LLM converting it to the relevant query syntax. This would be a great move forward in terms of democratizing data. But again, you will always also need the human in the loop to validate the information.”
But yes, we’re not there yet. In fact, in some ways LLMs are proving a distraction.
“Too many people are seeing LLMs as an all-round solution,” Joe says. “We need to realign and put the focus back on the specific problem at hand. In the end, LLMs may be part of the solution but we shouldn’t be leading with it. We need time to figure out that sweet spot.
“But we’ll only be able to do that with quality data management. Then we’ll be ready to take on the next tech breakthrough."
Contributor

AR
Ann-Marie Roche
Senior Director of Customer Engagement Marketing
Elsevier
Read more about Ann-Marie Roche