Your data can shape a better future
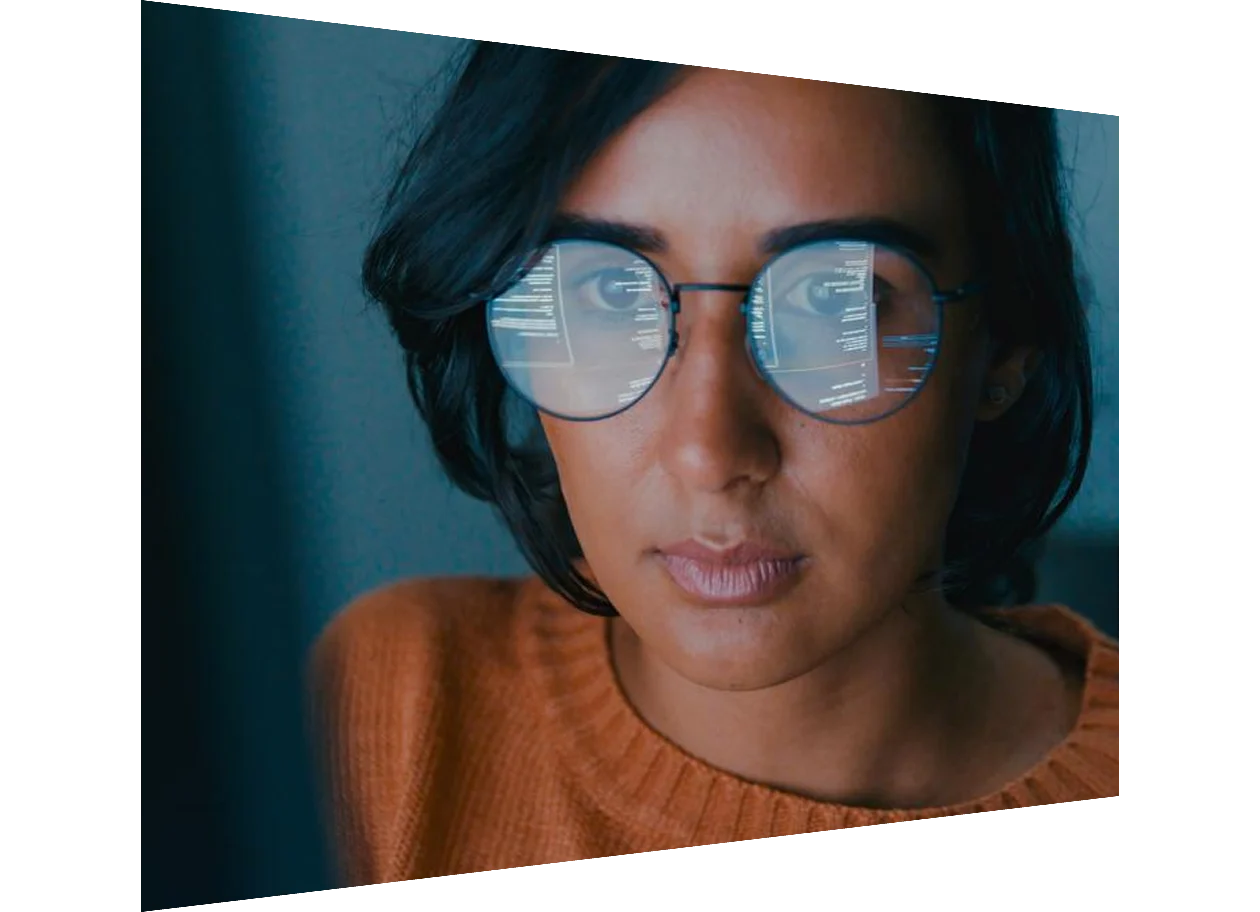
When data is shared responsibly, it empowers other researchers to build on those findings, reusing and reinterpreting information for further insights. It strengthens the foundation for replication building confidence in research and by increasing visibility and citations, researchers receive the recognition they deserve for their hard work. We’re making data sharing seamless and accessible for the research community to enhance the impact every researcher can make, and ensure their work is celebrated.