Mobile AI helps health workers deliver prenatal care in rural India
October 15, 2021
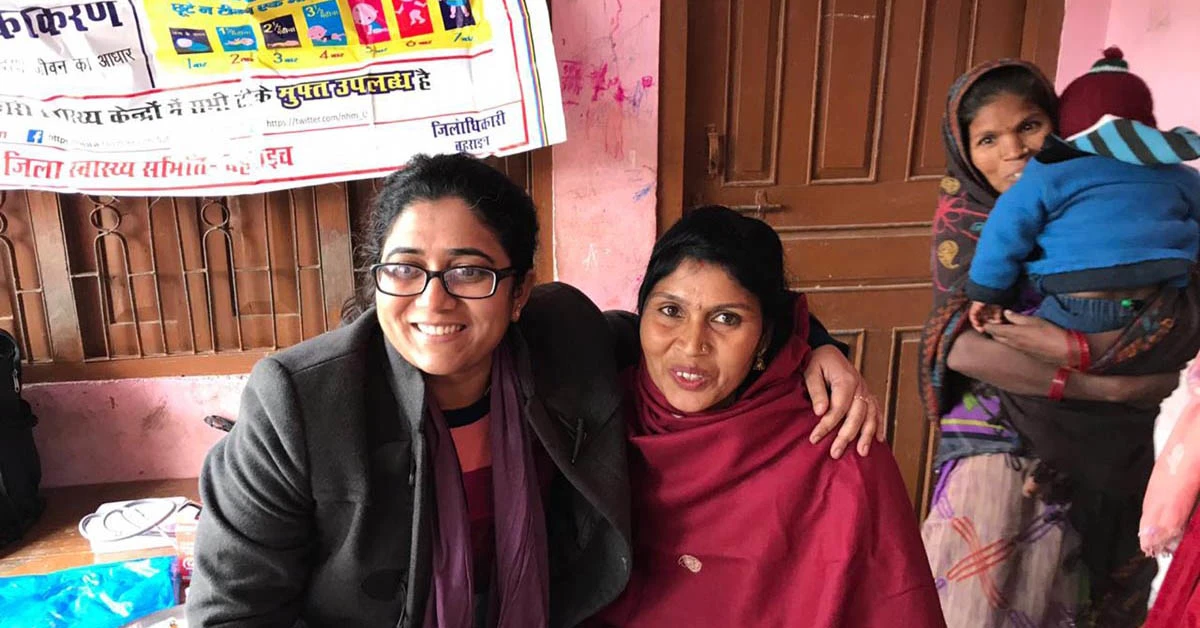
Photo by Meera Sani Gupta, Elsevier
To improve prenatal care in rural India, a partnership is equipping nurse midwives with Arezzo’s AI clinical decision support system Pictured above: Most healthcare in remote regions of India is delivered by accredited social health activists (ASHAs). Here, Co-Investigator Dr Aparna Sharma, Additional Professor in the All India Institute of Medical Sciences (AIIMS) Department of Obstetrics and Gynaecology, is with an ASHA worker at an Anganwadi, where consultations are given to women and children.
In India, making healthcare delivery inclusive means ensuring that people have access to the kind of care and information that can help save lives. That’s not always easy given the lack of availability of physicians in some of the country’s most remote villages. But it was a challenge that needed to be addressed.
A partnership between Elsevier and the Lady Hardinge Medical College and All India Institute of Medical Sciences (AIIMS) opens in new tab/window in Delhi found a way to combine the availability, dedication and empathy of the country’s auxi auxiliary nurse midwives with the AI clinical decision support system Arezzo.
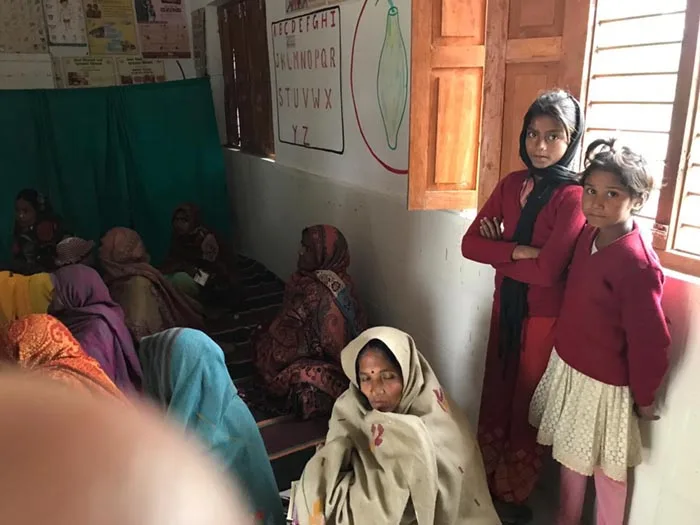
Women wait for a consultation with an auxiliary nursing midwife (ANM) at an Anganwadi — a place for providing health consultations for women and children in Indian villages. (Photo by Meera Sani Gupta, Elsevier)
In 2015, India accounted for 15% of global maternal deaths, and the country continues to have high maternal mortality. Only 58.6% of the mothers in India had a prenatal check-ups in their first trimester, partly due to the scarcity of qualified physicians in remote villages. Non-governmental organizations such as Piramal Swasthya Medical Research Institute (PSMRI) opens in new tab/window deliver healthcare in rural India, including outreach clinics and telephone triage services, which can meet the challenge of a geographically diffuse population. To standardize the way they were capturing data, PSMRI started to build their own electronic medical record system.
As Dr Robert Dunlop, Clinical Director for Clinical Solutions at Elsevier, recalled:
As part of that, PSMRI issued a request for information for clinical decision support tools. That opened up a conversation about using our sophisticated AI system Arezzo, which is designed to more closely replicate the way a clinician approaches clinical problems.
That conversation evolved into a series of initiatives to inform the Government of India Department of Health and Family Welfare about Elsevier’s capabilities. These efforts culminated in the RELX Government Affairs team participating in a UK-India Healthcare AI Workshop in 2018, which led to a pilot project funded by the UK-India Healthcare AI Catalyst program (HAIC). Elsevier won the competitive tender to deliver a showcase AI solution in India working alongside Lady Hardinge Medical College opens in new tab/window. The aim was to improve the knowledge of frontline non-physician health workers with a mobile, adapted version of the Arezzo Clinical Decision Support System, and it had a specific focus on prenatal care and care of newborns and children under 5 years of age.
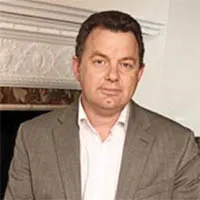
Robert Dunlop, Ph.D
That conversation evolved into a series of initiatives to inform the Government of India Department of Health and Family Welfare about Elsevier’s capabilities. These efforts culminated in the RELX Government Affairs team participating in a UK-India Healthcare AI Workshop in 2018, which led to a pilot project funded by the UK-India Healthcare AI Catalyst program (HAIC). Elsevier won the competitive tender to deliver a showcase AI solution in India working alongside Lady Hardinge Medical College opens in new tab/window. The aim was to improve the knowledge of frontline non-physician health workers with a mobile, adapted version of the Arezzo Clinical Decision Support System, and it had a specific focus on prenatal care and care of newborns and children under 5 years of age.
The team gave participant healthcare professionals Android-based tablets with 4G internet connectivity and a pre-installed instance of the AMRIT (Accessible Medical Records via Integrated Technologies) Android-based mobile app, developed by PSMRI as the implementation partner and integrated with Arezzo. With the support of a team of expert obstetricians and pediatricians, Elsevier transformed the Government of India standard treatment guidelines into computer-interpretable guidelines in English and Hindi; these were provided to healthcare workers. It allowed them to use patient data to receive real-time care guidance and action-oriented advice for individual conditions.
Robert explained the reasoning behind the initiative:
The majority of care in these remote locations is delivered by accredited social health activists (ASHAs) and auxiliary nursing midwives (ANMs) – women who live locally and have had some clinical training from the government.
The ASHAs and ANMs work from the village’s central register stored in AMRIT to follow up with women and their children. This ensures that the beneficiaries are seen on a regular basis. In addition to providing prenatal care, they check childhood development milestones and do some triage work if children are sick.
“It’s a lot of responsibility,” Robert said, “and they weren’t always equipped with the information and resources they needed.” Consequently, only an estimated 20% of patient visits adhered to standard treatment guidelines before the Elsevier solution was introduced. “That’s partly because people forget, partly because of pressure, but also because these are laypeople who have been trained up, and it can be hard for them to feel empowered to take action or intervene.”
The importance of that empowerment was echoed by Manju Puri, Director Professor and head of Obstetrics and Gynaecology Department at Lady Hardinge Medical College, and principal investigator for the project. She recalled what first caught her attention:
For me, it was very interesting in terms of improving and standardizing (the care) patients receive by empowering the healthcare providers. Because they’re using a mobile device, it means they’re not wresting with paper guidelines — their access to information was much easier and more dynamic. (Information) comes up at the right time in what can be quite a pressured environment.
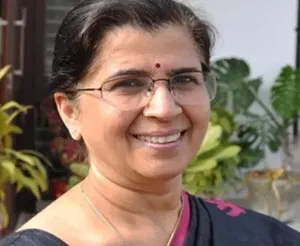
Manju Puri
The dynamic nature of the information is critical. A linear guideline can work as long as there are zero complications and every patient presents in the same way with that one condition – which, as any healthcare professional knows, is very often not the case. In prenatal care, multiple guidelines are involved covering risk assessment, anemia management, immunizations, and birth preparedness for example.
That’s where the particular type of AI that Arezzo delivers is especially relevant; it’s known as a declarative artificial intelligence — or an “expert system.” As Robert explained:
It’s designed to mirror the way experts think. It is used to look after patients with multiple diseases. In order to do that, you would have information about the conditions in your head, and from all these different pieces of information, you draw down those bits that are relevant to the patient, pull them together and personalize them.
Declarative AI can replicate that thought process through the blending of clinical content, reasoning, AI, decision recommendations, and explanations in a way that is distinct from traditional branching models. It was this element that attracted Dr Varinder Singh opens in new tab/window, Professor of Pediatrics at Lady Hardinge Medical College, to the project. He explained:
There have been a lot of fanciful ideas about what AI would do in health, suggesting a machine could learn gradually and then give you an accurate diagnosis. We’re a long way from that kind of science fiction. But we knew Elsevier had AI, and we were curious to see whether it could work.

Varinder Singh, MD, FRCPCH
With timelines being tight, Varinder described “taking the plunge” with Arezzo and evaluating how it would help bring reliable, actionable information to the auxiliary nurse midwives of rural India:
We realized that this was about the rapid digitization of what was on hardcopy, and that digitization was intensive. It wasn’t merely something translated onto a user interface – it was interactive. It used AI to select which guidelines might be useful depending on the particular patient or set of triggers.
Varinder explained that diseases have “a limited vocabulary,” meaning that the same set of five or six symptoms could be indicative of a number of diseases:
How do you differentiate? You need an algorithm that is sensitive enough and rapid enough to pick up cases from the field to be referred to for specialist care, but which doesn’t lose specificity to the point where everybody gets referred.
With a useful AI tool in place, the program was rolled out into the community. Initially, the frontline workers were a little apprehensive about using a tool that initially seemed quite elaborate, Manju recalled. But seeing people put it to use was inspiring:
That made me feel very happy – it was so good to see them getting used to the technology. You really got the sense that they were feeling more in control of the situation, having the required knowledge at their fingertips. They were very happy about it, and it was one of the key things that made me feel that this could be taken forward.
Once the frontline workers were out in the field with the technology, the results were remarkable. Equipped with these tools, the frontline workers detected that 223 of 603 women had high obstetric risk. They referred 15 women for urgent care and 31 women for non-urgent care. Additionally, 78 children – 8% of those seen as part of the initiative – have been recommended for immediate referrals.
Of the people using the tool, 98% agreed that it gave them more information on what to advise patients, 96% agreed it helped them remember all diagnostic procedures to be advised, and 93% agreed that it helped them administer the right treatment and the right drug at the right dose. Varinder concluded:
I think this was a real shift for these frontline healthcare workers, as they really saw they could play an even bigger role in helping their communities. I remember one person saying that they’d identified some high-risk pregnancies as well as identifying children who needed further intervention, sending them for further care.
It was a real sign that they had started believing in their own ability to make a huge difference.
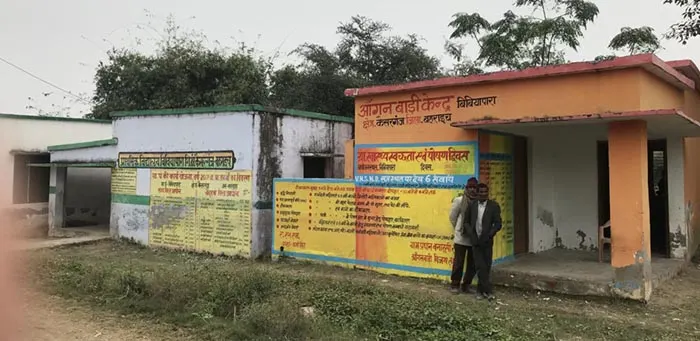
An Anganwadi outreach clinic (Photo by Meera Sani Gupta, Elsevier)
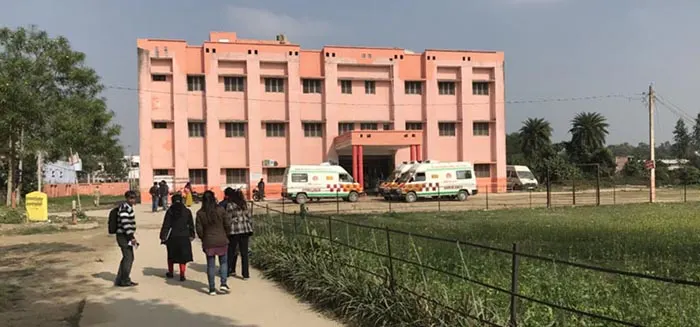
A secondary-level referral hospital for mothers and children. (Photo by Meera Sani Gupta, Elsevier)