Research Data Management: Everything your institution needs to know
Discover the world of research data management, from funder policies to available tools and resources.

Research organizations need to carefully manage all the data resulting from their efforts throughout its lifecycle. This means everything from collection to storage, analysis, sharing and archiving or disposal should receive focus and a best-practices approach.
Collectively, this is research data management (RDM), a process that allows data to fulfill its role as the collective output of researchers' efforts. High-quality RDM is necessary to advance the state of science and discovery today and to reinforce the integrity of results, as well as to help organizations collaborate across fields and borders. It's also helpful for giving individual researchers and institutions the recognition they deserve for their efforts.
Getting RDM right often comes down to an organization's selection and effective usage of specialized technology tools. The right solutions for this purpose will reflect the unique and essential nature of managing the research data life cycle.
A table of contents:
What is research data management?
Types of research data
Key elements of research data management
Key data-sharing policies from funding agencies
What role do academic libraries play in research data management?
Further learning for academic librarians
What are the best practices for research data management?
FAIR Principles:
Research Data Framework (RDaF)
How do you develop a data management plan?
The phases of a data management plan
How do you store research data securely?
How do you keep research data confidential?
What is the most secure way to store data?
How do Yyou share and showcase your data?
Why is sharing research data important?
Why is collaborating on research data important?
Why is showcasing research data important?
What is research data management?
RDM is a set of practices designed to keep data accessible and secure. Organizations practicing effective RDM are those that are preserving all the key research output resulting from their research efforts, while archiving or disposing of content in line with their individual fields' requirements and guidelines.
The practices and guidelines associated with RDM are designed to ensure data is available, secure and trustworthy. Content collected and kept in accordance with accepted RDM principles should be comprehensible, reusable, citable and reproducible, strengthening not just a specific research project but the institution and field as a whole.
Rather than being one specific thing, the term "research data" incorporates every piece of research output associated with a project. This can encompass a wide variety of media, across formats. Examples include:
All data sets collected as part of the research.
Researchers' notes (whether physical or digital).
Test results.
Recordings taken during the process.
Software used in the research.
Images and other visual files.
Key elements of research data management
The practices associated with RDM extend from end to end, beginning before a given project launches and lasting throughout the open-ended lifespan of the data. There should be methodical steps followed at every specific part of the process, divided up into functional areas such as:
Planning: Research organizations must plan their RDM efforts in advance, and they should be integrated into their overall strategies.
Collection: Standardized, reliable methods for data capture are essential parts of RDM. These must encompass all relevant formats used by researchers.
Storage: An intuitive and effective repository is necessary for keeping necessary data and making it available to researchers within the organization and outside of it.
Organization: Researchers need to know what content is being stored, as well as where it's kept and how it's being used, which is accomplished with dedicated technology tools.
Security: Research data must receive adequate protection to preserve its integrity, guard against loss and ensure unauthorized parties don't access or tamper with it.
Preservation: Making necessary data available and easily discoverable for future use is important, as is deleting information with a limited lifespan in a timely manner.
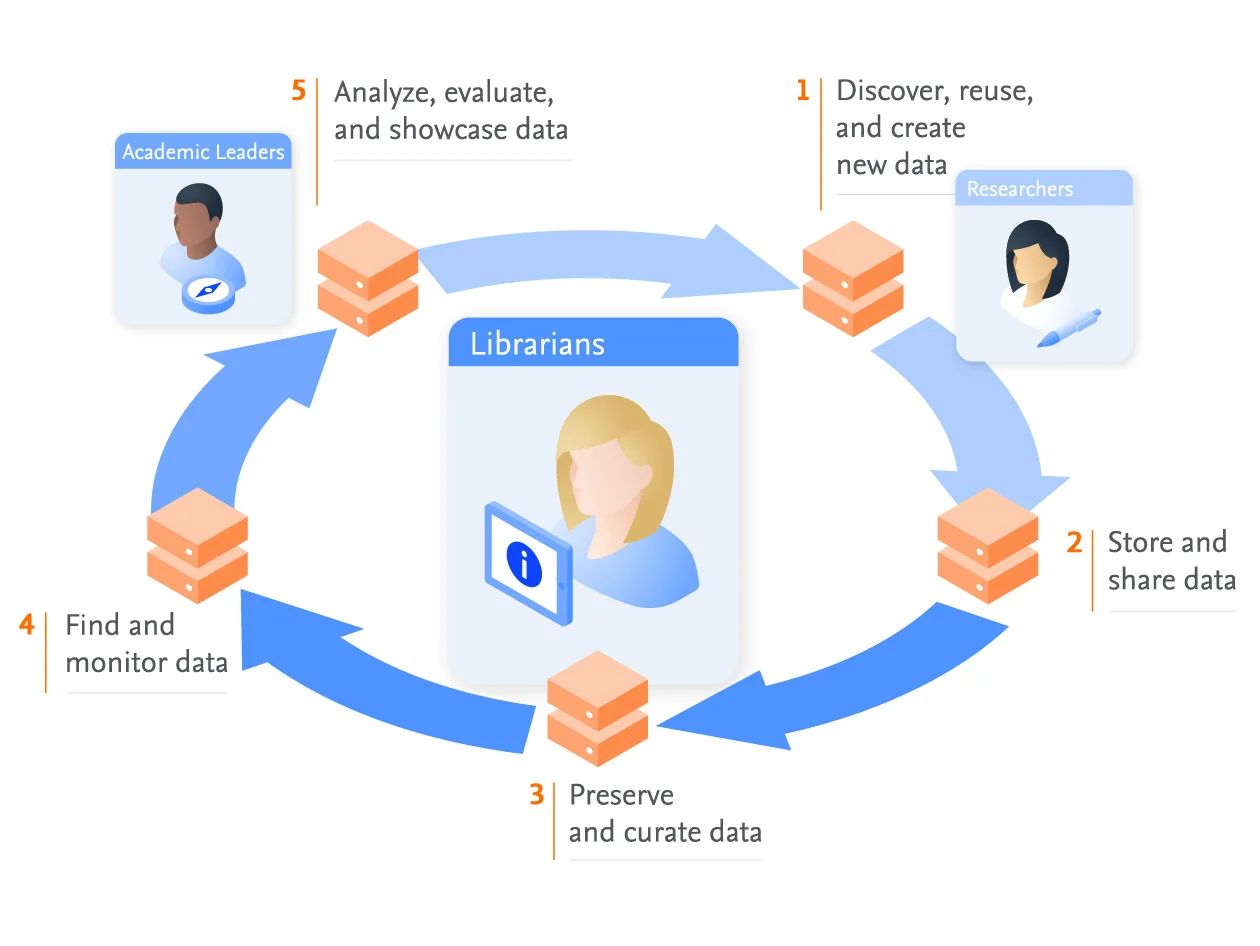
Research data management lifecycle
Key data sharing policies from funding agencies
Government agencies and other funding organizations have a large degree of influence on how RDM is practiced, with their policies shaping the best practices followed by organizations within their verticals. While there are countless RDM policies across regions and fields for researchers to follow according to their own needs, it's worth inspecting some examples:
European Commission open data, software and code guidelines: opens in new tab/window The European Commission guidelines dictate the standards a study needs to meet for submission to Open Research Europe. These include storing datasets in approved repositories, licensing the data's reuse, removing sensitive, identifiable details, sharing software and code, making the data align with FAIR Data Principles and more.
NIH policy for data management and sharing: opens in new tab/window This policy establishes standards for research published or funded by the National Institutes of Health (NIH), and also "emphasizes the importance of good data management practices." The NIH data management rule governs all aspects of data creation, storage and usage, recommending how to keep and present data, how long to retain it and where to share it for optimal coordination with other research organizations.
NASA biological and physical sciences open data: opens in new tab/window The National Aeronautics and Space Administration policy serves as a useful example of individual organizations' open data guidelines. The policy is aimed at making datasets as open and accessible as possible, to increase the impact of data collected during the agency's experiments. It's designed to fit with NASA's ongoing adoption of FAIR Data Principles.
Organizations and partnerships within the RDM field have spent the past few years implementing their own tools and frameworks to meet and exceed sharing and accessibility requirements. These include the Transparency and Openness (TOP) guidelines for standardized journal citation laid out by the Center for Open Science. As with many government-mandated frameworks, FAIR Data Principles often form the backbone of these systems.
While accepted guidelines make it clear that high-quality data management is a compliance matter, and necessary for funding, they also underline the role of RDM in strengthening collaborative and iterative experimentation in general. It's worth exploring the many reasons to adopt practices in greater detail.
What are the benefits of research data management?
An organization performing advanced RDM is putting in work to establish the utility and trustworthiness of its own data. This has both immediate and long-term benefits, both within the context of the research that generated the data and in the overall progress of the organization's sector.
RDM is also part of a research group's obligation to perform its work in a responsible way, and to empower collaboration with other organizations. The scientific method is based on reproducibility and clear reporting of results, and RDM aligns with these pillars.

Generalized benefits of RDM include:
Helping researchers draw accurate conclusions from their own data: While RDM is essential for sharing data between groups, its utility starts much earlier. Researchers will find it easier to work through their own data when it's been collected and stored in line with the principles and standards of RDM.
Complying with requirements for funding and publication: The compliance aspect of RDM comes from the agencies and other funding organizations that publish and support research. While it's important to determine what specific practices are warranted in any case, there are common throughlines, such as the use of FAIR Data Principles.
Boosting the credibility of research outcomes: The transparency associated with high-quality RDM helps researchers present their conclusions to the scientific community. Results from clearly notated, repeatable experiments, supported by well-documented, verifiable data, are ideal for pushing entire fields of study forward.
Assisting with securing future funding: Beyond laying down guidelines for how organizations must report their results, funding organizations will also want to verify that their funds have led to useful outcomes. Having transparent, provable results from research can encourage this effect.
From applying for funding to engaging with other researchers, RDM can convey benefits at numerous stages in the research life cycle. In today's institutional landscape, good practices in this area are a necessity for organizations eager to distinguish themselves as leaders in their specific fields.
What role do academic libraries play in research data management?
It's natural for an institution's academic library to act as the locus for RDM best practices. This is true for two reasons, as described opens in new tab/window in a peer-reviewed Open Access Library Journal paper:
First, academic librarians are often the individuals within an organization who have the most direct experience with RDM principles from their own training and past work. These are the go-to point people for adding new data management technologies and practices.
Second, the academic library will typically have strong relationships with various stakeholder groups throughout the organization. This will help the RDM solutions proposed by the library take hold and encourage continued collaboration.
With this ideal positioning, research libraries can become the coordinators for the various efforts that go into increased RDM focus, from purchasing software to training relevant staff. Having such a centralized authority behind the RDM project can benefit an organization overall, with clear guidance for the program.
Further learning for academic librarians
Academic librarians who want to learn more about RDM adoption have a variety of options. Educational resources that can reveal deeper insights include:
What are the best practices for research data management?
What defines a great RDM program? While every institution will have its own specific use case for RDM based on its field, size and subject matter, there are goals and general practices that apply widely and can serve as guiding stars for RDM adoption.
Perhaps the most reliable universal framework in these cases is the FAIR Principles opens in new tab/window, commonly adopted in a RDM context and referenced by numerous funding organizations in their requirements. These principles, first published in 2016 and with wide applicability in the world of research data collection, storage and sharing demand that data be:
FAIR Principles:
Findable: In FAIR terms, being findable means data is easy to locate, whether researchers are looking through resources manually or using an algorithm. The need for machine discoverability means data should be paired with metadata.
Accessible: Accessibility goes hand-in-hand with being findable but is different. This means once the user has found the data, they need to be able to understand how to retrieve, authenticate and authorize it.

Interoperable: Interoperability means data can integrate with other data sets, and is compatible with storage, processing and analysis methods. Using standardized systems, language and vocabulary is a key part of ensuring interoperability.
Reusable: Falling in line with the scientific method and its focus on reproducible experiments, the FAIR Principles require data to be richly described, have a clear usage license, meet community standards and have accessible provenance.
Technology platforms designed with research data in mind can make it easy for researchers to share their results in line with FAIR Principles. The Mendeley Data FAIRest Datasets Award program highlights research groups following this approach to presenting data.
Research Data Framework (RDaF)
It's worth noting that there are frameworks beyond FAIR designed to achieve similar ends, and with the support of major institutions. For example, the U.S. National Institute of Standards and Technology's Research Data Framework (RDaF) opens in new tab/window is meant to support trustworthy data sets in both natural and social science contexts.
The RDaF is designed to be customizable for individual organizational needs. It's intended to change the RDM culture at an organization when implemented, with a focus on the research data lifecycle. Under the RDaF, these lifecycle stages are:
Envision.
Plan.
Generate/acquire.
Process/analyze.
Share/use/reuse.
Preserve/discard.
Treating RDM with this level of focus and intentionality across the entire existence of information resources is a valuable practice for any organization in the field.
How do you develop a data management plan?
Achieving high-level success in RDM doesn't simply happen. Institutions need to build specific plans for research projects to both align all internal stakeholders and establish their credentials to funding organizations — for instance, the NIH now requires an approved Data Management and Sharing Plan opens in new tab/window for research it conducts or funds.
A data management plan is a living document that lays out what data will be collected, how it will be used and what the organization will do to store it and maintain it. Data management plans must be locked in early in the course of a project, before any data is collected and while approval is underway. There are numerous opens in new tab/window templates opens in new tab/window and examples opens in new tab/window available opens in new tab/window from institutions across the spectrum of subject matter to help researchers guide their own efforts and stay in line with funding organizations' requirements.
The exact nature of a finalized data management plan will differ depending on the data being collected — its origin, purpose, sensitivity, complexity and other factors will determine the appropriate form for the plan.
While the documents are important, they don't necessarily have to be complex. By explaining in simple terms how data will be collected, used, stored, shared and published, the plan makes it easy for any internal or external stakeholder to quickly answer questions about the related data.
The phases of a data management plan
One main consideration with a data management plan is comprehensiveness. Though the document is completed early in a project, it prescribes how data should used through the whole life cycle:
Preparatory phase: Rules should be in place for how data will be collected and kept before research begins, so there are consistent best practices observed throughout.
Data organization: Storing, organizing and curating data are activities that determine the direction of the project and establish its reliability.
Analysis and dissemination: Analyzing, sharing and retaining data in line with best practices helps research drive the discussion forward in its field.
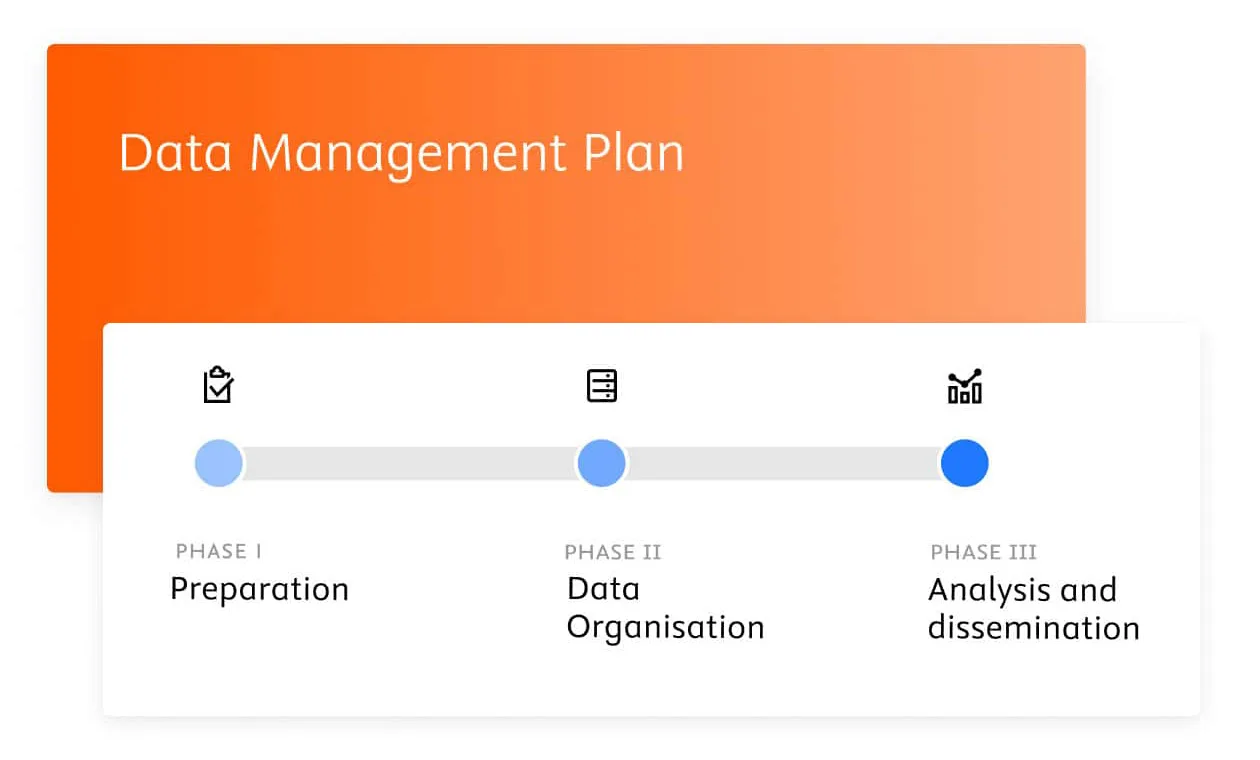
How do you store research data securely?
Data security doesn't just mean one thing in any digital context, including RDM. Rather than protecting against accidental access, inadvertent loss, hacking or destruction in a natural disaster, stakeholders have to guard against all these issues and more.
How do you keep research data confidential?
The confidentiality and overall protection of research data is essential for an organization's compliance status and reputation. Effective and comprehensive data security combines many methods, including:
Password protection and encryption: Digital files should only be kept on systems that require strong passwords for access and support encryption to prevent unauthorized access.
Physical defenses for physical media: From detachable digital devices to paper records, today's researchers still store data in physical forms. These must be kept in secure areas.
Need-to-know data access policies: To prevent the inadvertent leak of confidential information and simplify security, it's important to give data access to a minimal group.
Strong data destruction methods: If sensitive data is set to be destroyed at the end of an approved timeline, the process should be comprehensive, leaving no identifiable traces.
Employee training and oversight: Any researcher or worker who deals with research data should understand their responsibilities in protecting it.
Data backups and disaster recovery: As with important corporate data, scientific data should be stored in systems that can survive natural disasters, power loss and other incidents.
What Is the most secure way to store data?
There is no single best way to store, access and retain research data. The ideal method will depend on an institution's circumstances and the conditions set by regulators and its funding organizations. Options for today's researchers to choose from include:
Cloud computing: While the cloud may not work in all cases (for instance, if regulations demand data be kept on researchers' own systems), it does have advantages. For example, cloud resources receive frequent security updates and are protected by large institutional owners.
Physical and on-premise systems: While the responsibility for protecting on-premise resources falls on their owners, they do bring the benefit of complete control. Furthermore, internal systems can be "air gapped" in extreme cases, with no connection to the internet for potential intruders to use.
Hybrid solutions: Many organizations today rely on a combination of on-premise solutions and cloud resources, with each system called upon for some kinds of data storage.
How do you share and showcase your data?
In today's research environment, sharing data is often required and even more often encouraged. This means an RDM effort would be incomplete without methodologies for allowing third parties to discover and sort through research data.
The actual process of making data available alongside publication will, in the most straightforward option, involve placing the data in a repository and linking to it within the article so it's easy to access.
Why is sharing research data important?
Sharing research data helps researchers build their own reputations — they can take credit for their meticulous work in collecting and analyzing the content and may receive more citations and publication rates. Fellow researchers also benefit, as they can build on the work and take the line of inquiry further, using the principles of open science to advance knowledge as a whole.
Why is collaborating on research data important?
Collaboration and the sharing of complete, reliable data sets allows researchers to make progress on complex problems without duplicating work that has already been done. RDM practices ensure the data is trustworthy and verifiable enough for this collaborative approach.
Why is showcasing research data important?
Making data available is a key principle in today's research space, and can help increase the trust in both the specific research in question and science as a whole. Efforts are underway to make research increasingly comprehensible in light of ever-greater public attention, and RDM has a part to play in these projects, delivering reliable scientific data sets with clear provenance.
What are the best research data management tools in 2024 and beyond?
An organization's choice of technology platform can determine how easy a time that institution has in implementing both the general principles and the day-to-day practices of high-quality RDM. There are a few traits every chosen technology offering should share. For example, the U.S. federal government suggests opens in new tab/window that data repository tech tools should include:
Authentication of data submitters, matching people with the digital objects they add.
Long-term technical sustainability and plans for ongoing management.
Security and integrity that conform with industry standards.
Rather than counting on one technology tool to handle every aspect of RDM, sufficiently large and advanced research organizations will likely operate several technologies. These cover every aspect of the data life cycle.
Elsevier's offerings handle these diverse requirements and deliver a comprehensive RDM experience include:
Digital Commons Data: This institutional data repository offers numerous key RDM functions — storing, managing, publishing and preserving research data sets. It is usable by researchers themselves as well as administrators and dedicated curators. It supports real-time collaboration, dashboard-based analysis and flexible, long-term storage.
Pure: As a Research Information Management System (RIMS), Pure allows institutions to oversee their research activities. The solution assists with discovering funding opportunities, managing publication, collaborating with other organizations and more. It's an indispensable tool for processing information about an institution's research performance.
Mendeley Data: This free, secure, cloud-based research data repository is designed for widespread use by research teams. Uploading content to Mendeley Data helps it become part of a worldwide network of easily shared and cited data, in line with numerous institutions' RDM policies.
Commit to research data management
Once you've acknowledged the need for RDM, it's time to set your institution up with a technology platform that will allow it to live up to its potential and ideals. This process may involve asking some questions about your tech needs and the ideal responses:
The lists of recommended and certified data repositories opens in new tab/window across fields and subject matter are long, and those categories don't always match. Investigating in depth to find the ideal RDM option for a specific institution can be challenging for this reason, but is a necessary undertaking to prepare your organization for its immediate and long-term future.
With your RDM objectives becoming clearer, you can discover your ideal customized research data management solution using the most trusted tech solutions in the sector.