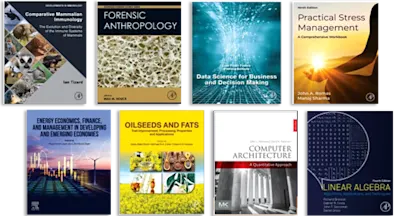
Machine Learning for Planetary Science
- 1st Edition - March 25, 2022
- Imprint: Elsevier
- Editors: Joern Helbert, Mario D'Amore, Michael Aye, Hannah Kerner
- Language: English
- Paperback ISBN:9 7 8 - 0 - 1 2 - 8 1 8 7 2 1 - 0
- eBook ISBN:9 7 8 - 0 - 1 2 - 8 1 8 7 2 2 - 7
Machine Learning for Planetary Science presents planetary scientists with a way to introduce machine learning into the research workflow as increasingly large nonlinear datasets… Read more
Purchase options
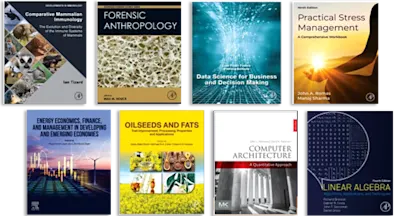
Machine Learning for Planetary Science presents planetary scientists with a way to introduce machine learning into the research workflow as increasingly large nonlinear datasets are acquired from planetary exploration missions. The book explores research that leverages machine learning methods to enhance our scientific understanding of planetary data and serves as a guide for selecting the right methods and tools for solving a variety of everyday problems in planetary science using machine learning. Illustrating ways to employ machine learning in practice with case studies, the book is clearly organized into four parts to provide thorough context and easy navigation.
The book covers a range of issues, from data analysis on the ground to data analysis onboard a spacecraft, and from prioritization of novel or interesting observations to enhanced missions planning. This book is therefore a key resource for planetary scientists working in data analysis, missions planning, and scientific observation.
- Includes links to a code repository for sharing codes and examples, some of which include executable Jupyter notebook files that can serve as tutorials
- Presents methods applicable to everyday problems faced by planetary scientists and sufficient for analyzing large datasets
- Serves as a guide for selecting the right method and tools for applying machine learning to particular analysis problems
- Utilizes case studies to illustrate how machine learning methods can be employed in practice
Graduate students and researchers working in planetary science, especially data analysis and planetary missions
- Edition: 1
- Published: March 25, 2022
- Imprint: Elsevier
- Language: English
JH
Joern Helbert
MD
Mario D'Amore
MA
Michael Aye
HK