GenAI: The double-edged sword revolutionizing drug discovery
2025๋ 3์ 25์ผ
์ ์: Ann-Marie Roche
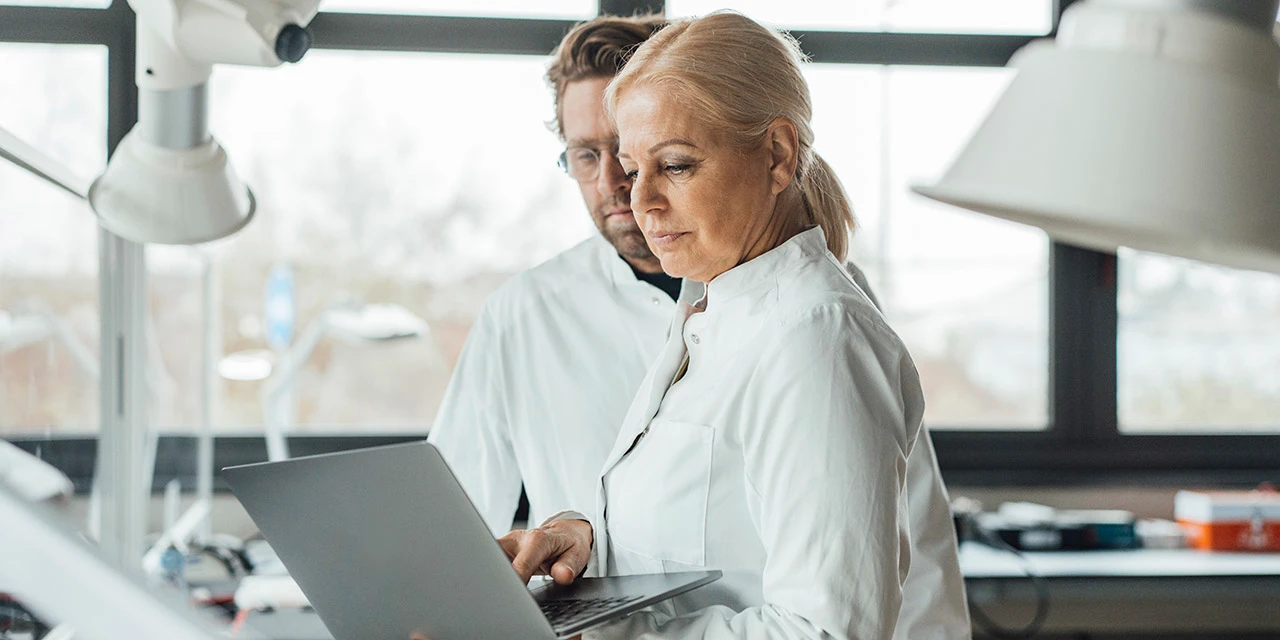
Westend61 via Getty Images
How do you harness GenAIโs transformative power for R&D while safeguarding scientific integrity? Experts share their insights and success stories.
We all want to apply AI to improve patient outcomes. But how?
โItโs about passion,โ says Dr Ivan Krsticย ์ ํญ/์ฐฝ์์ ์ด๊ธฐ, Senior Director of Life Sciences at Elsevier, as moderator of the webinar Generative AI: Transforming Knowledge and Drug Designย ์ ํญ/์ฐฝ์์ ์ด๊ธฐ. โAnd I believe all of us here share this passion for harnessing computational advances to transform drug discovery and improve patient outcomes.โ
Of course, with passion come practicalities โ and much room for improvement. Current drug discovery approaches typically require three to five years and โฌ50 million to โฌ100 million per new candidate, and they still have only a 10% to 20% success rate.
So itโs easy to understand why researchers in the life sciences want to adopt AI solutions to streamline this process. But first, the industry needs to face some fundamental challenges: data quality and availability, integration with existing systems, regulatory uncertainties, talent and expertise requirements and scalability concerns.
โI believe all of us here share this passion for harnessing computational advances to transform drug discovery and improve patient outcomes.โ

IK
Ivan Krstic, PhD
Elsevier์ Senior Director of Life Sciences
Opportunities on one side, challenges on the other
Itโs tricky, but the effort is more than worth it, according to the two guest experts on the webinar โ the first installment of the Foundations for Effective AI webinar series for 2025. While AI tools offer tremendous potential โ such as the ability to explore vast chemical spaces beyond traditional libraries โ they should be viewed as aids to human expertise rather than replacements.
โAI is not magic,โ stresses Dr Brice Hoffmannย ์ ํญ/์ฐฝ์์ ์ด๊ธฐ, Principal Scientist and Head of Computational Chemistry at the innovative French AI company Iktosย ์ ํญ/์ฐฝ์์ ์ด๊ธฐ. โItโs a tool that will help you decide what to make โ and thatโs already good enough.โ
โAI is not magic. Itโs a tool that will help you decide what to make โ and thatโs already good enough.โ

BH
Brice Hoffmann, PhD
Iktos์ Principal Scientist, Head of Computational Chemistry
On the future of scientific research
According to Dr Arash Hajikhaniย ์ ํญ/์ฐฝ์์ ์ด๊ธฐ, Research Team Leader at VTT Technical Research Centreย ์ ํญ/์ฐฝ์์ ์ด๊ธฐ of Finland, who studies and assesses AIโs positive and negative impact, AI is already reshaping the role of computational chemists in modern research.
On one fundamental level, GenAI can open doors and unite a community once limited by language barriers. Arash highlights how Generative AI democratizes science by breaking down language barriersย ์ ํญ/์ฐฝ์์ ์ด๊ธฐ โ a crucial advancement considering that 95% of the worldโs population are not native English speakers.
But while GenAI may work to democratize science, you must also work to protect its integrity. Arash warns of an emerging โvicious circleโ where generative AI is filling up the internetย ์ ํญ/์ฐฝ์์ ์ด๊ธฐ and โpolluting scientific discourse and literature.โ
"We are faced with the critical task of addressing this self-sustaining loop of misinformation," he says, pointing to a trend where AI is now being used to peer-review papers that may be AI-generated. โThis creates a potentially dangerous echo chamber that could further undermine scientific integrity.โ
In short, researchers must be vigilant and apply strategies to identify AI-generated content, and the peer review process must evolve to address these challenges.
On emerging technologies
Meanwhile, GenAI has already proven its worth. Arash cites the practical solution of combining Large Language Models (LLMs) with knowledge graphs. Through its ability to โunderstandโ context and make semantic connections, this integration helps researchers navigate the ever-growing volume of scientific literature more effectively while minimizing GenAIโs proclivity to hallucinate when not trained on the correct information.
For instance, when searching for โbreakthroughs in AI for cancer diagnosis,โ the integrated system can prioritize recent publications while identifying relevant connections across research papers, datasets and clinical trials.
Meanwhile, Brice offers his companyโs efforts as a compelling case study on how AI-driven approaches can help pharmaceutical companies accelerate their Design-Make-Test-Analyze (DMTA) drug development research cycle.
Iktosโs end-to-end drug discovery platformย ์ ํญ/์ฐฝ์์ ์ด๊ธฐ integrates AI-driven design and synthesis prediction to cut development time in half. Brice shares a concrete example of AI-assisted drug design for a C1s inhibitorย ์ ํญ/์ฐฝ์์ ์ด๊ธฐ. The Iktos system generates molecules that achieve a 10-fold improvement in binding affinity through strategic molecular structure optimization.
โBy combining multi-parametric optimization with AI-driven retrosynthesis, we can design molecules that are very fast to synthesize and test,โ Brice explains. โWith this rapid turnaround, we can accelerate the DMTA cycle.โ
An emerging trend such as autonomous AI agents, where an AI can cast its net across a variety of AIs and data sources, will only work to further transform โ and accelerate โ research methodology and decision-making. Improved robotics will undoubtedly also accelerate DMTAโs โTโ for testing.
However, we must also work to understand how these increasingly complex systems generate their outputs.
On validation and quality control
โWe need to ensure all innovations follow human-centric design principles and are validated by peer review,โ Brice emphasizes, bringing the discussion back to Arashโs opening concerns about scientific integrity. Systems also need to include their own checks and balances. For instance, with the Iktos two-pronged approach, one AI works to make a molecule, and another evaluates whether the suggestion is feasible โ an approach that fortunately can also filter for greenness and cost.
Arash also recommends that researchers not limit themselves to one LLM, pointing out that there are 15,000 available, each trained for different purposes. However, he stresses the importance of data quality, illustrated by the 10:10 clock phenomenonย ์ ํญ/์ฐฝ์์ ์ด๊ธฐ: When asked to create a clock, LLMs invariably set it to 10:10 because thatโs how clocks appear in their training data (i.e., the internet). As Arash notes:
โGarbage in, garbage out. The LLMs are bound to the data they are trained on โ so look at that data carefully!โ

AH
Arash Hajikhani, DSci
VTT Technical Research Centre, Finland์ Research Team Leader
On implementation and ethics
Regarding overall implementation, Arash believes the key to success lies in individual responsibility:
We are a community benefiting from this solution. If we don't use it responsibly and pollute, we will suffer from that same solution.
Arash also warns that over-reliance on these tools could impact researchersโ cognitive capabilities. โWhen we write, we also think,โ he notes, suggesting that outsourcing too much of the scientific process to AI could potentially diminish human analytical skills: โI try to use them as mentors to elevate my capabilities, for instance, with my writing. Instead of asking to proofread, I ask the LLM to give me hints and tips so I can learn gradually along the way.โ
The discussion concludes with a powerful reminder from Ivan:
โWe need to be good citizens, taking responsibility for the responsible use of AI tools and ensuring we publish only whatโs true and validated.โ

IK
Ivan Krstic, PhD
Elsevier์ Senior Director of Life Sciences
The path forward
To summarize, while AI presents opportunities and challenges for the scientific community, success lies in finding the right balance: leveraging AIโs capabilities while maintaining human oversight, accelerating processes while ensuring accuracy, and embracing innovation while preserving scientific integrity.
As research becomes increasingly AI-enabled, this balanced approach will be crucial in realizing AIโs potential to transform scientific discovery and improve patient outcomes โ further fueling the passion that drives researchers in the life sciences community forward.
Learn more
To dive deeper into how Elsevier helps discover new materials and drives innovation by applying trustworthy data, watch Foundations in Action: Applying Elsevier Chemistry Datasets in R&D workflowsย ์ ํญ/์ฐฝ์์ ์ด๊ธฐ.
Related stories
๊ธฐ์ฌ์

AR
Ann-Marie Roche
Senior Director of Customer Engagement Marketing
Elsevier
Ann-Marie Roche ๋ ์ฝ์ด๋ณด๊ธฐ